Building a Learning Culture: The Problem of Organizational Knowledge
Product managers are well positioned to turn organizational learning into a competitive advantage.
Every time I watch a teammate walk out the door for another opportunity, I wonder how much of our organization’s knowledge leaves with them. Surely an organization can “know” more than what’s inside each employee’s head. But how is this knowledge acquired? Where is it stored? Is it written down in documents, or is it tacitly woven into a team’s culture?
These questions are especially pertinent to product managers. Think about a product manager identifying the winning variant of an A/B test. Given enough of these tests, the PM begins to build an intuition for what tends to work. But does the organization share these data-driven intuitions?
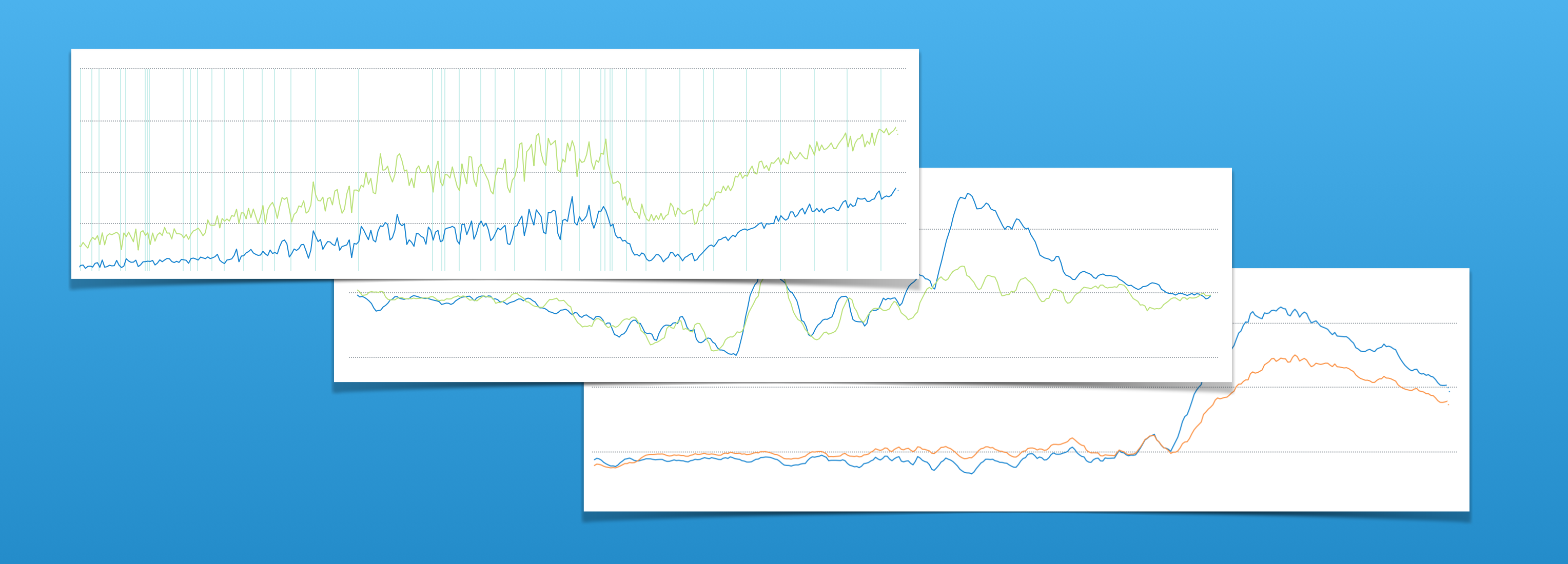
I believe that organizational learning can be a source of operational effectiveness and even a competitive advantage. Further, product managers are in a unique position to enable such learning due to the data-driven nature of our work. My team of product managers has been experimenting with a process to translate our product experiments into shared organizational knowledge. This is the first in a series of posts that will describe how we approach building our team’s knowledge base.
Memes: What We Think We Know
If we accept the premise that organizations can learn, we should also consider whether organizations can learn incorrectly. In other words, how do teams develop false beliefs?
When making product decisions, I’ve learned to be very skeptical of one form of belief, which I’ll call a meme. I use “meme” in the original sense of the word, coined by Richard Dawkins in his 1976 classic The Selfish Gene. Dawkins defined meme as “a unit of cultural transmission” and elaborated:
Just as genes propagate themselves in the gene pool by leaping from body to body via sperms or eggs, so memes propagate themselves in the meme pool by leaping from brain to brain via a process which can be called imitation. (192)
In other words, a meme is a particularly sticky and repeatable idea that may not necessarily be true. Product memes take such forms as:
- “We know that users hate X because when we tried it a few years ago, we got a lot of complaints.”
- “Users value X. When we added X to our signup flow caused a Y% increase in retention.”
- Adding unnecessary taps to a flow will result in drop-off.
These types of claims could be true. But since memes are detached from their original context, it’s hard to know whether they—or their supporting data—can be trusted. In order to increase our confidence, we’d want to ask questions:
- Can you describe the experiment design that led to this conclusion?
- How exactly was X executed? What did the screen look like?
- In this context, what measure of retention are we talking about?
These sorts of questions might seem like nitpicking, but without them the data-driven emperor is wearing no clothes. Instead of building organizational knowledge, we risk stifling it. A better approach involves critical thinking inspired by scientific principles. In his 1974 Caltech commencement address, Physicist Richard Feynman described the essential ingredient that differentiates science from pseudoscience: “The idea is to try to give all of the information to help others to judge the value of your contribution; not just the information that leads to judgment in one particular direction or another.”
We’ve arrived at the first problem that must be solved in order to build data-driven organizational knowledge: When insights are detached from their original context and supporting data, we can’t be sure of what we think we know.
PR Statistics: More Stylized than Analyzed
Memes often take hold in organizations unintentionally, but sometimes they are seeded with a very particular purpose. Think of the last great pitch you heard from a Product Manager—or anyone else in your organization. More than likely their presentation contained a few striking pieces of data that helped make their point. Here’s an example of the type of data I often use to emphasize how well a given feature is performing: Users that use feature A have a X% higher rate of success compared to our average user.
For any product professional reading this, it should be obvious that this is a classic case of “correlation does not imply causation.” (Feature A is likely a symptom of high engagement, not a cause.) While this is a fair critique, I would still argue that stylized facts or “PR statistics” as I like to call them have their place, provided we remember not to confuse them with “real statistics.”
- PR Statistics: Numbers used to tell an artful story or formulate hypotheses.
- Real Statistics: Numbers used to inform product decisions with confidence.
Product management is both art and science, and there are times when deviating from scientific purity is the right decision. Perhaps you are making a speculative bet where hard data isn’t available. Perhaps you don’t have time to run an experiment. In these situations, product managers might relax their analytical standards and use PR statistics. But for the sake of organizational learning, we must be crystal clear about the limitations of such an approach.
We can take this idea even further: Not only should we steer clear of “PR statistics” when building organizational knowledge, we must fully engage with botched experiments as well as negative results. When an A/B test comes back with disappointing results, it’s tempting to quietly move on to the next hypothesis. But in doing so we miss an opportunity to learn. The combination of “PR statistics” and concealing bad news can lead to a culture of what one writer has dubbed “Success Theater,” which is the antithesis of organizational learning.
This brings us to our second problem to be solved: While data is more plentiful than ever, it is often more stylized than analyzed, failing to meet the higher standard required for organizational learning.
Even if our methods of analysis are held to a high standard, the craft of product management is about more than statistics. Great product managers develop intuitions and theories of why certain things tend to work. And yet I’ve read countless reports that conclude with statements like “When given the option between Treatment A and Treatment B, users preferred Treatment A.” In most cases, we could say much more.
Aristotle wrote in Metaphysics that “art (or craft) arises, when from many notions gained by experience one judgmentabout similar objects is produced.” In other words, we truly understand something only when we develop a unified theory that explains many observations. Aristotle’s description captures the type of organizational learning we aspire to create. We want a team that shares a common theory of user behavior that predicts what tends to work and why.
Of course, not every experiment will clearly point to a reason why. And some experiments will venture into new territory, leaving the team without the benefit of the “many notions” that naturally hone our intuitions. Additionally, I sometimes fear that creating a culture with very high analytical standards has the unintended consequence of making product managers feel like they shouldn’t make speculative leaps to account for their results.
But without some degree of speculation, we can’t develop our theories of what works and why. We’ve now arrived at our third problem to be solved in order to build organizational knowledge: While a rigorous approach to analysis is required to build organizational knowledge, it can sometimes inhibit the creative speculation that sparks genuine insight.
Next in this Series: Building the Barn.
Sam Rayburn, a long serving Texas congressman and Speaker of the House, once said “Any jackass can kick a barn down, but it takes a carpenter to build one.” In that spirit, understanding the problems of organizational knowledge is not the point of this series. What really matters is constructing a system that solves these problems. The rest of this series will be devoted to the system we’ve developed to foster a learning culture. Below is a preview of some of the key elements:
- To move beyond memes, we have standardized the written format of our product experiment reports.
- To raise our evidentiary standard above “PR statistics,” we instituted a multi-phase review and discussion processto scrutinize methodologies, analyses, and conclusions.
- To move beyond the numbers and develop theories, we’ve instituted an explicit “no bullshit” policy and learned to relentlessly ask ourselves the question “What did we really learn?”
- To push the knowledge outside the product team, we’ve organized a regular series of cross-funtional workshops to review data and develop theories of user behavior.
In the next few posts in this series, I’ll dive deep into each of these topics and detail how we are building our barn. As with any educational pursuit, ours is constantly evolving and changing. But I believe the underlying principles of our process can give others a head start on building their own learning cultures. We may not be able to eliminate the shock—and the cost— of a valued teammate’s departure, but we can make our barn more resilient to unexpected storms.
I hope you’ll be back to read more.